Urban public space commonly accommodates freight delivery operations through on-street (un)loading zones (LZ). Given the scarcity of public space and its demand by various users, city authorities face the challenge of effectively managing LZ by understanding freight curbside needs and utilization. Despite the popularity of technological solutions and enforcement practices among policymakers to capture curbside dynamics, an open and promising research field is focused on designing analytical frameworks that guide LZ decision-making processes.
This has inspired researchers to introduce the concept of Smart Loading Zones (SLZ), which involves integrating technology and data analytics in planning and managing LZ in a responsive and user-oriented manner. In addition to proposing a conceptual approach for studying SLZ, this paper employs data analytics tools to enhance decisions related to LZ network design, with the City of Vic (Spain) serving as a case study.
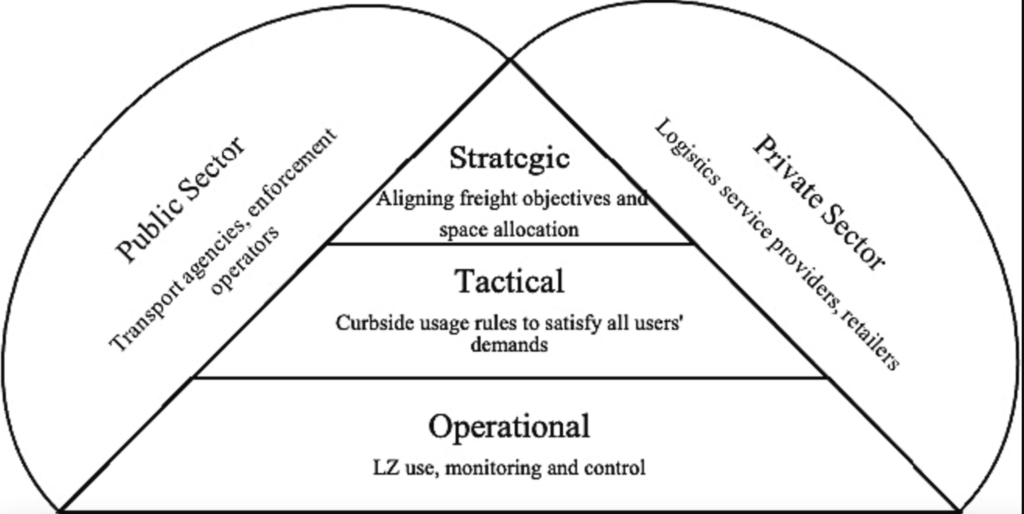
The strategic level encompasses LZ network design, which involves determining the quantity, location, and number of parking stalls for loading zones. It also entails technological assessments related to stationary devices for LZ management, such as sensors, cameras, parking meters, or other fixed devices. From a private sector standpoint, the strategic level involves allocating transport resources based on freight demand, access regulations, and the availability of loading zones in specific city zones.
The tactical level pertains to decisions concerning duration limits, pricing, dimensions of loading zones, and the implementation of mobile technology by various loading zone users. In the private sector, this level involves determining fleet allocation, considering factors like vehicle type, size, and technology that align with traffic policies and customer requirements.
At the operational level, the public sector establishes enforcement schemes and initiatives for information-sharing among users and evaluates operations’ impact on traffic, the environment, land use, and logistics efficiency. On the private sector side, decisions are made regarding routing under constraints such as LZ availability and freight demand.
Machine learning techniques and integer linear programming are applied to determine the optimal LZ number, location, and service assignment based on the coordinates of establishments, walking distances, and freight demand. The case study results demonstrate how optimizing LZ’s number, location, and size significantly improves occupation levels, increasing from 18 to 80 percent while freeing up curbside space for other users.
Allocating LZ to establishments within a 75-meter walking distance enhances service coverage. The paper recommends further development of methods and tools for SLZ at tactical and operational decision levels in future studies.